This is a continuation of a series of posts looking at December seasonality in the stock market. The previous post is here, and the goal of this series is to both examine the seasonal tendency and to share the thought process.
Today, we’re going to do something a little big dangerous. Let’s do it first, then we’ll talk about why it’s dangerous.
In my previous posts on this topic, we’ve seen that there does seem to be a December effect in stocks. (And maybe also a November effect… and a September effect…) Let’s ask another question: is the tendency different in bull markets or bear markets? Take a look at the table below.
It seems that Santa might be afraid of the bear! Decembers were only up 56% of the time in bear markets. On the other hand, Santa seems to really like flat markets—December was up 83% of the time in flat markets.
Now, we should ask some more questions. The first question you should be asking is “how did we determine bull and bear years.” While I don’t disclose all the details, I can tell you this: it’s a purely mathematical tool that is designed to replicate the experience of a trader trading through that time period. In other words, it’s not just a simple return on the year—it also looks at daily trends and volatility.
It’s also not critically important, and you can easily categorize the years yourself if you want. For reference, my tool gives us, for the DJIA:
- Bull years: 1897, 1899, 1904, 1905, 1908, 1909, 1915, 1919, 1922, 1925, 1927, 1928, 1929, 1933, 1935, 1936, 1943, 1945, 1950, 1951, 1954, 1955, 1959, 1963, 1964, 1971, 1975, 1976, 1983, 1986, 1987, 1989, 1995, 1996, 1997, 1999, 2007, 2013, 2017
- Bear years: 1900, 1902, 1903, 1907, 1910, 1911, 1913, 1914, 1917, 1920, 1921, 1923, 1930, 1931, 1932, 1934, 1937, 1940, 1941, 1942, 1953, 1957, 1960, 1962, 1966, 1969, 1970, 1973, 1974, 1977, 1981, 1984, 1988, 1990, 2000, 2001, 2002, 2008, 2009
Here’s a graphic (click for full size) showing the distribution of red and greens for the different slices:
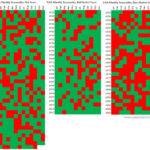
One potential issue with this classification is that it is based on end of year. A trader trading in December would have information (i.e., a pretty good guess whether the year was a bull or bear year), but a trader in January would not have that same insight. (For other types of analyses, we can use a rolling window to mitigate that, but this work was done on calendar years.) Just something to think about, and since we’re focusing on December it’s at least consistent.
Potential issues
Here’s where things get a little bit less clear. Each time we take a “cut” through the data, we are in danger. Why? Because doing so potentially allows us to fit the question to the data very well. We will get a result that looks very impressive, but that has no predictive power.
An example would be (and you’ve seen them all over the internet) something like this: What if the S&P is up on a Tuesday in the 3rd or 4th week of the month, and the month is up between 1% and 2%, and last week was a down week, and Monday opened up from Friday’s close on high volume? When we see this (which has occurred 4 times since 1532) the market has closed up 100% of the time…
It’s obvious in that case that someone was probably up all night trying different things. You can create an impressive backtest by trying things, keeping the things that work, and then adding more conditions. However, it just won’t carry through to the future.
What is more insidious are the cases where you can’t tell. Maybe I show you a great test that only has one condition, but I was up all night trying many different things before I found the ONE THING that really worked. This is a form of data mining, and you have no idea, without intimate familiarity with the process, how much of it happened.
And, it gets even worse. If you’re an active market participant, you are already doing these slices through the data without realizing it. Just thinking about what to test and then executing a test, if you’re a trader who is very “in touch” with the markets, risks this kind of overfitting and data mining.
So what’s the answer? To my mind, there’s a middle ground. Some degree of this refinement is necessary, and we have a word for it: learning.
This is what happens when we understand how a market moves. (Yes, right out there at the edge of this discussion, some really interesting topics are lurking—machine learning and genetic algorithms. We’ll revisit those another day…) So, just be aware, and be careful. If you find yourself needing four or five “at bats” to get the stats you want from the market, you’re probably in trouble. If you get an answer after that kind of work, it’s probably not a good answer.
There’s also the question of statistical significance. Take a look at the Decembers in the table at the top of this article again. Is 83% really (like, actually, really-really, for sure…) different than 56%? Probably, but is 56% really different from 72%, or do we think it could just be luck and noise in the data? How sure are you of that answer? How about 72% and 74%? Really different, or not?
There’s one more post in this series, and we’ll take a look at daily returns and think about how to answer that question.
Oh, and I’ll also show you the real Santa Claus…